How AI Is Revolutionizing Log Analysis in Security Operations
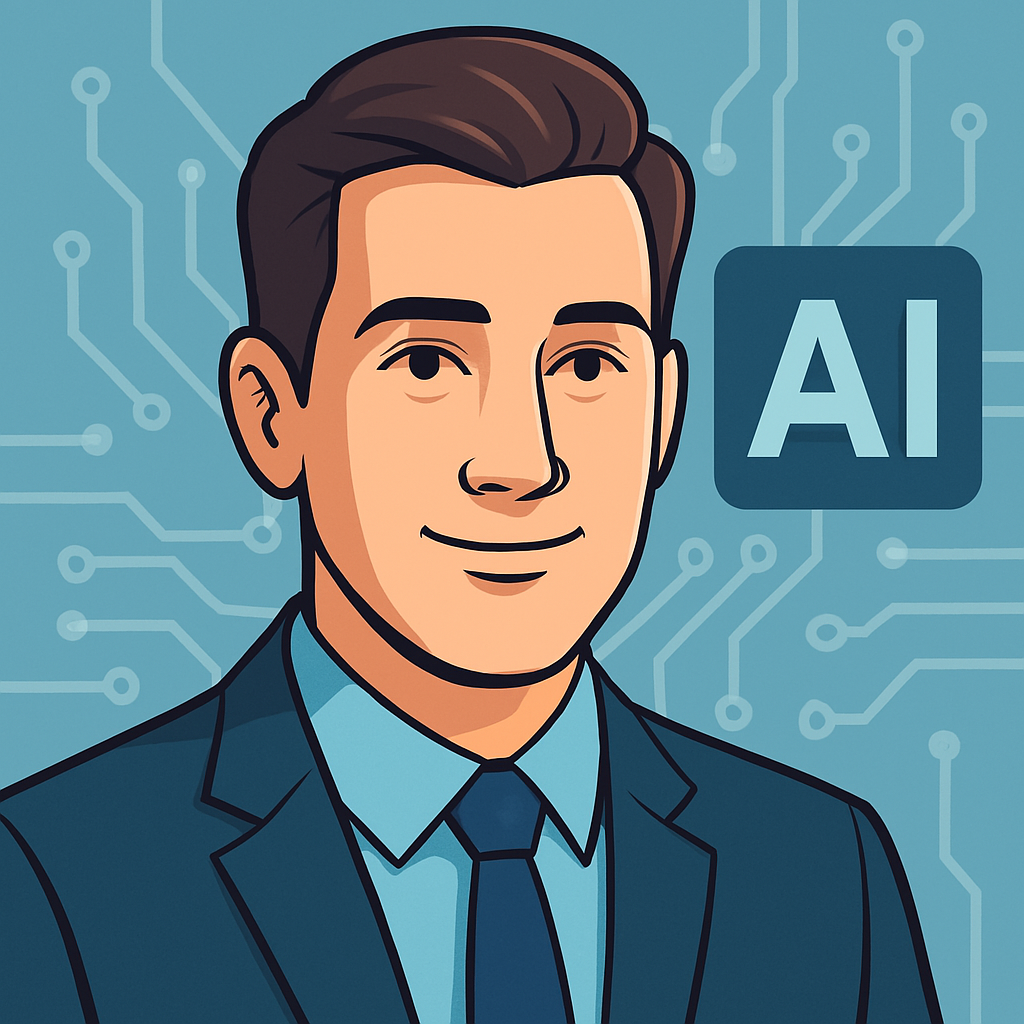
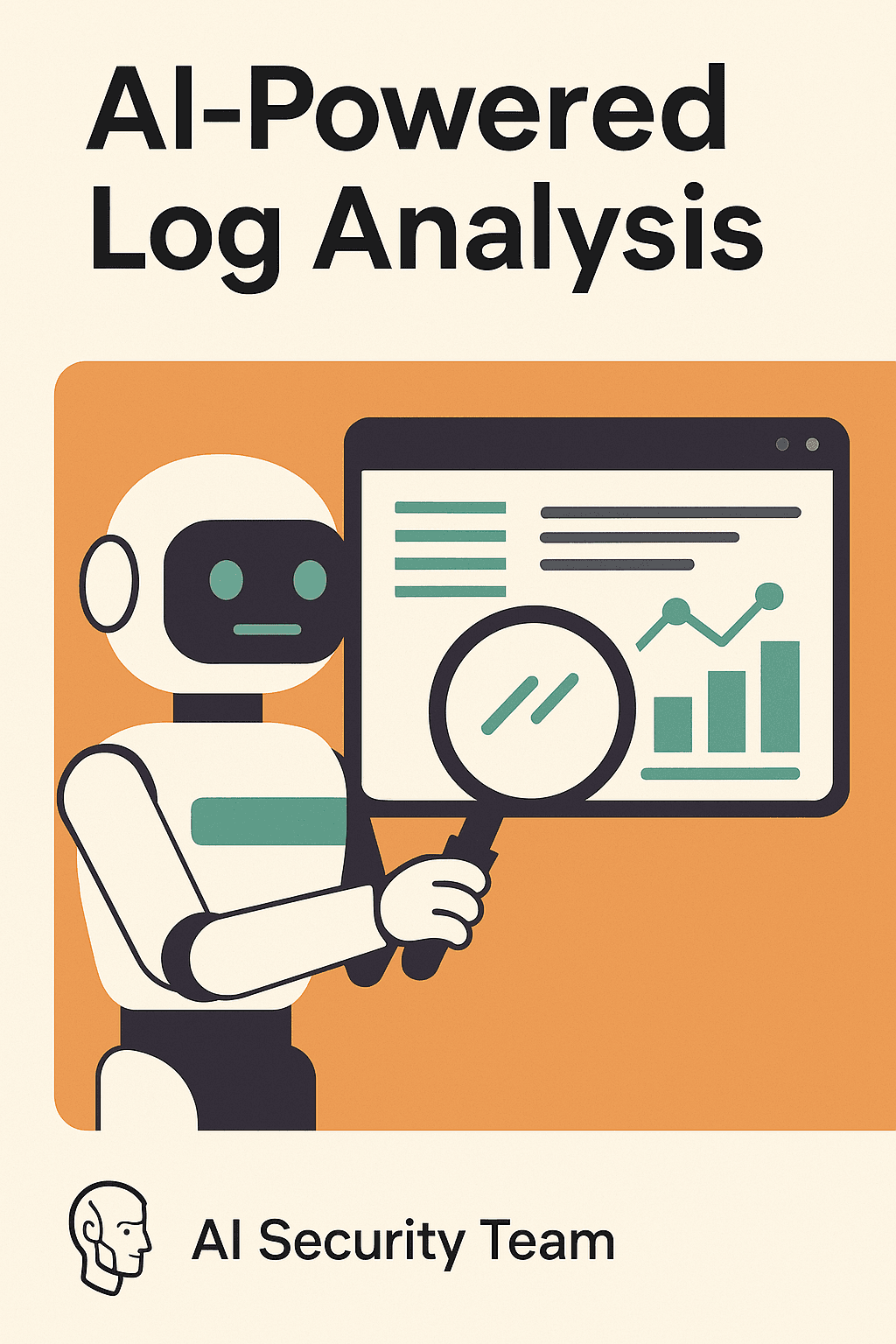
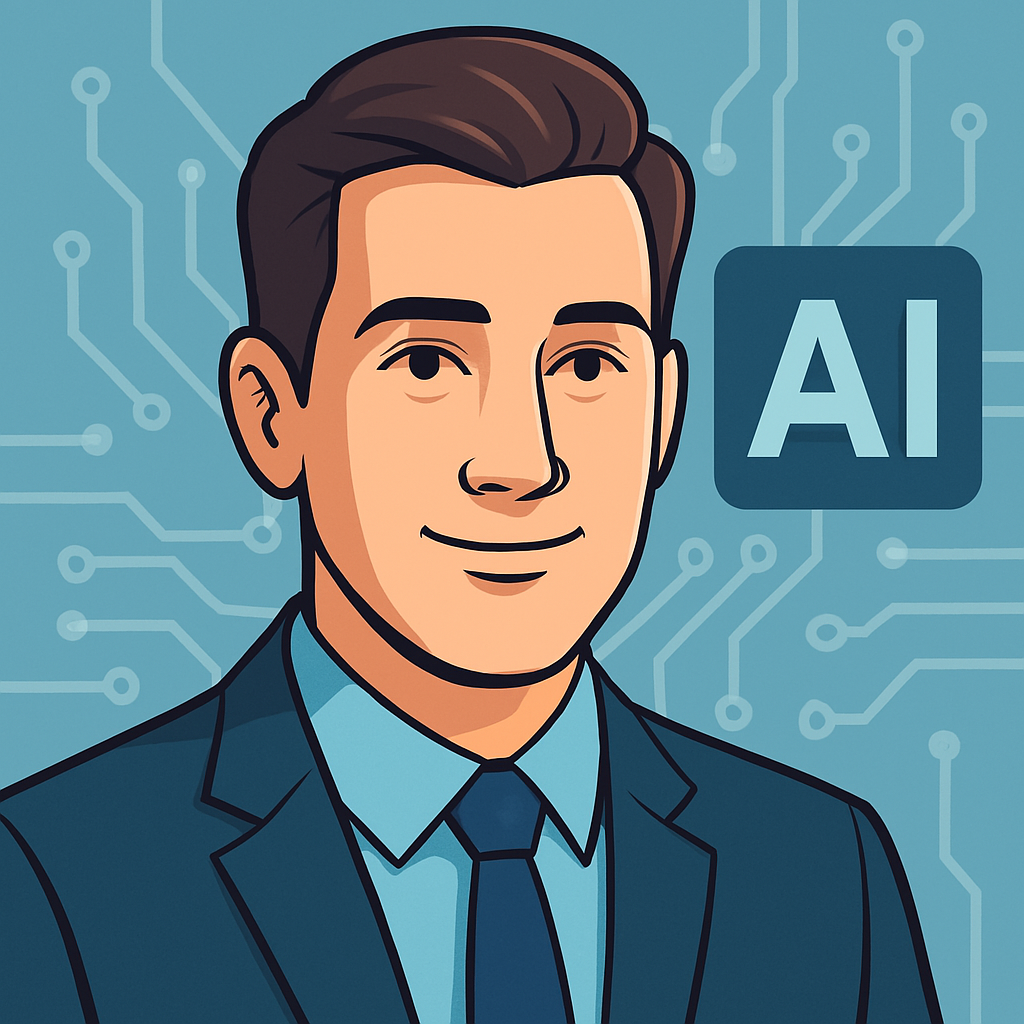
Log analysis is one of the most fundamental activities in cybersecurity — and also one of the most difficult to scale. Security teams rely on logs to detect attacks, troubleshoot incidents, and meet compliance obligations. But with modern infrastructure producing billions of log events daily, humans alone can’t keep up.
AI is changing that.
By applying machine learning (ML) and large language models (LLMs) to log analysis, security operations centers (SOCs) are gaining unprecedented visibility, speed, and accuracy in identifying and responding to threats.
The Log Analysis Challenge
Security logs come from a wide variety of sources:
- Firewalls
- Cloud services (e.g. AWS CloudTrail, Azure Monitor)
- Endpoint detection tools (EDR/XDR)
- Authentication systems (SSO, VPN)
- Applications and databases
The challenges?
- 🔎 Too many logs to review manually
- 😵♂️ Inconsistent formats and fields across systems
- 🧩 Lack of context between events from different sources
- ⏱️ Delays in finding root causes or detecting anomalies
This leads to missed threats, long investigation times, and overworked analysts.
Where AI Makes a Difference
AI supercharges log analysis by handling volume, identifying patterns, and surfacing meaning.
1. Machine Learning for Anomaly Detection
Unsupervised ML models can:
- Learn “normal” behavior from historical logs
- Detect statistically significant deviations
- Cluster similar outliers to reduce alert noise
For example, an AI model might flag:
- A user logging in from an unusual IP or location
- A service account making abnormal API calls
- A spike in failed login attempts over a short period
These signals often indicate early-stage attacks like credential stuffing or privilege abuse.
2. LLMs (e.g., ChatGPT) for Log Interpretation
LLMs can help make sense of complex logs by:
- Translating raw log entries into plain English
- Identifying suspicious patterns based on context
- Summarizing entire log sessions
Example:
Raw log:
[auth] failed login attempt for user admin from 10.2.4.8 on port 22
LLM interpretation:
“An unauthorized SSH login attempt was made to the ‘admin’ account from internal IP 10.2.4.8. This may indicate lateral movement or privilege escalation.”
This is especially useful for Tier 1 analysts and incident responders who need speed and clarity.
3. AI-Powered Correlation Across Sources
AI can help correlate log events across multiple tools:
- Match authentication logs with firewall logs and file system access
- Detect lateral movement that spans hosts, cloud services, and timezones
- Reconstruct attack paths based on behavioral patterns
Some security platforms use graph-based AI models to build visual timelines of attacker behavior — saving analysts hours of manual effort.
4. Real-Time Alerting and Enrichment
AI-enhanced log systems can:
- Automatically enrich logs with threat intelligence (e.g., IP reputation)
- Prioritize alerts based on business risk or asset criticality
- Feed insights into SIEM/SOAR tools for faster remediation
Platforms like Splunk, Elastic, and Microsoft Sentinel now offer AI-based detection rules, automated enrichment, and pre-trained models for known attack patterns.
Tools That Apply AI to Log Analysis
- Elastic AI Assistant (uses LLMs to explain logs in plain English)
- Splunk Machine Learning Toolkit (train models on your log data)
- Logz.io AI-Powered Alerts
- Panther Labs (Python-based detection-as-code with ML support)
- OpenAI API + scripts for custom log summaries
Caution: Where Human Oversight Is Still Needed
While AI is powerful, it must be carefully tuned. Watch for:
- False positives due to unusual but legitimate behavior
- Model drift when your environment changes
- Hallucinated explanations from LLMs when logs are ambiguous
Always validate AI-driven insights with analyst review — and use feedback to retrain or fine-tune models over time.
Final Thoughts
AI is rapidly transforming how we interact with logs. What used to take hours of manual digging can now be completed in seconds — with better accuracy and less fatigue.
By pairing anomaly detection, correlation engines, and language models with experienced analysts, security teams can move from reactive investigation to proactive detection.
As logs keep growing, AI isn’t just helpful — it’s essential.